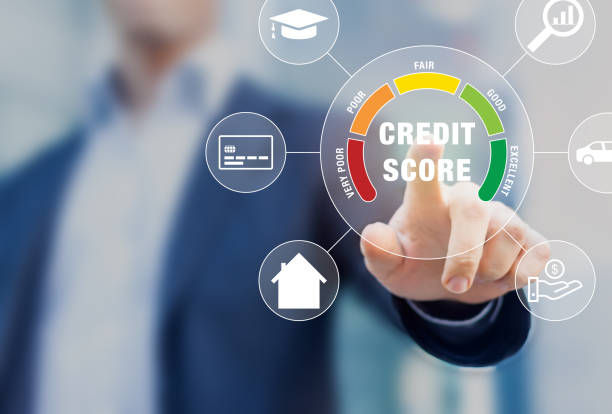
Credit Score Modelling
Constructed a credit scoring model, based on logistic multiple regression, for acceptance of credit based on five predictors namely age, dependent income, indicators of home ownership, self-employment and the number of notices of insolvency of an individual within a fixed time period.
This project aims at constructing a credit scoring model, based on a logistic multiple regression, for acceptance of credit based on five predictors namely age, dependent income, indicators of home ownership, self-employment and the number of notices of insolvency of an individual within a fixed time period. The consumer’s application outcome has been considered as the dependent variable. This project aims primarily at exploring the following:
I. General overview of the problem and explaining why logistic regression is suitable to study the data of the kind considered here.
II. Making a choice of minimal best model that fits the data with Justification.
III. Further discussions on the chosen model with sample scenarios.
The aforementioned exploratory and predictive analysis have been performed using R Programming language implemented in R Studio. Upon modelling and analysis, It was noticed that the important factors considered for approval of credit application were Age, Income, Number of Insolvency notices and the Employment status of the borrower ( i.e. Self Employed or Not). The highest direct relevance was observed with the income of the borrower whereas an inverse relationship was observed with other factors. It was also found that the home owner variable didn’t have any statistical significance in predicting the output.
Highlights
95 %
Significance Level Considered
7
# of Models Compared & Analysed
92.637
AIC of the Chosen Best Model
Project Gallery
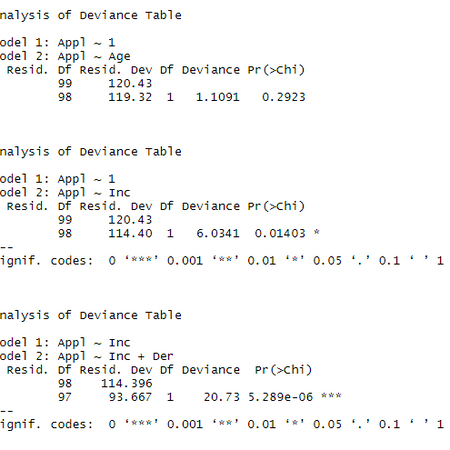